HUNTSVILLE – With the ever-increasing use of wireless communication technologies and cellular networks, radio frequency-based attacks such as “spoofing” have become a significant concern due to the privacy and security risks in military and civilian communication networks.
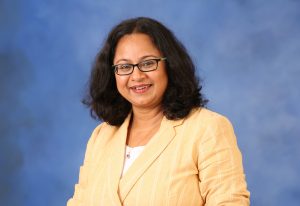
Spoofing is an attack where an adversary transmits RF signals coded to trick any RF communication receiver, such as those utilized by the Global Navigation Satellite System (GNSS), into accepting something as legitimate when in fact it’s not. For example, spoofing a GNSS signal could make a military drone fly in the wrong direction or lead a cargo ship into pirate waters.
Dr. Chaity Banerjee Mukherjee, an assistant professor at the University of Alabama in Huntsville, has won a $1 million Air Force Research Laboratory (AFRL) grant to develop frameworks for artificial neural networks that will help combat these attacks.
Artificial neural networks comprise a branch of machine learning algorithms inspired by the structure and function of the human brain, a type of mathematical model that, after being ‘trained’ on a given dataset, can be used to make predictions on new data. By simulating the way spoofing works, these artificial intelligence models can learn to better identify and defend against these incursions, protecting public safety and even national security.
“What excites me about this work is that it has real-world consequences, leading to breakthroughs in both military and civilian RF simulation-use cases,” Banerjee Mukherjee said. “The goal is to build AI models – artificial neural networks in this case – that can generate realistic RF data by modeling real-world environmental settings.”
GNSS systems encompass different types of satellite-based positioning, navigation and timing systems used globally that employ constellations of satellites to accurately determine their own location by measuring the distance to four or more satellites. Spoofing can trick the GNSS receiver into thinking it’s somewhere that it’s not, or at a different point in time, or both.
“An important task in the field of wireless security and RF forensics is that of emulation of an RF environment,” the UAH researcher said. “These emulations can be used to study the characteristics of these environments as well as studying attack surfaces to better understand RF security.”
The “attack surface” of an RF environment – a piece of software or communications network – involves the different points where an unauthorized user can try to enter data, extract data, control a device or corrupt critical systems.
Harnessing the power of AI to combat spoofing
To understand how to counteract these intrusions, the researcher will use a variation of neural networks called a Generative Adversarial Network (GAN) to produce a framework for improving RF simulations. A GAN consists of two parts: a generator that learns to generate realistic ‘faked’ data, and a discriminator that quickly learns to flag the data as fake.
“In our framework, the generator will act as a malicious GPS emitter that generates fake GPS data with the goal of forcing the discriminator to make a mistake, accepting the fake data as real,” Banerjee Mukherjee says. “The discriminator in turn aims to minimize the mistakes it makes in classifying real GPS data from fake ones. During the training phase, the decision of the discriminator is conveyed to the generator, which uses this information to fine tune its parameters to generate more realistic ‘fake’ data.”
He said it’s challenging to model RF transmissions using deep learning because of the underlying real-world physics involved, factors like the speed of propagation, wavelengths, environments and interactions of electromagnetic communications.
“With the advent of large-scale RF emitter deployments, it has become increasingly difficult to manually characterize the resulting RF environments,” Banerjee Mukherjee said. “Electrical engineers can accurately simulate them in the lab. However, such simulations are time consuming and expensive, so it’s important to be able to achieve these characterizations through automated means. The physics-based simulation system can be used to produce realistic RF simulations and replace existing hardware-in-the-loop simulation systems that are used for research and training of personnel involved with RF signal data.
“My goal is to achieve the same simulation accuracies as the electrical engineers using AI in a data-driven manner,” the researcher said. “Ideally, this data should be indistinguishable from real over-the-air data, using much lesser computational complexity. We plan to test our system by building accurate GPS simulations for a given region. We’ll also produce a multi-emitter RF fingerprinting and spoofer detection system that will be a direct consequence of training the discriminator in the GAN-based setting.
“The advent of deep learning and its successful application provides us a tool that has the potential to automate these tasks.”
Don’t miss out! Subscribe to our email newsletter to have all our smart stories delivered to your inbox.